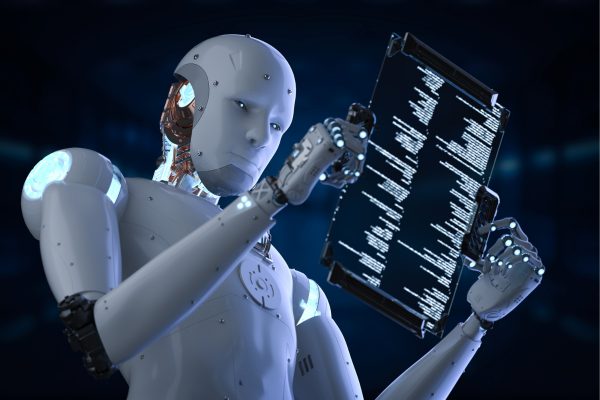
It’s hard to avoid hearing the term AI these days. Since the emergence of ChatGPT in November 2022 it has become the mainstream media’s most coined phrase. Straight-laced news shows would run opinion pieces talking to musicians or authors about the risks and challenges AI poses – the removal of creativity, plagiarism and the like, followed by a sample of a ChatGPT-generated poem in the style of…
Needless to say, the AI-generated output was plausible, but poor – all the right words, but none of the nuance that as humans we instinctively pick up on.
Another way to put it – AI is about as intelligent as a paperclip (some say).
So, what’s all the fuss about – is it all hype? Are we all taken in by software parroting stuff back to us on demand?
Basic Facts About AI
AI is not intelligence – the term itself is rather misleading. When computer scientists talk about computer intelligence equivalent to human intelligence (which has to be the measure by which we rank intelligence), they talk about AGI – Artificial General Intelligence – and that is a long, long way off. We should not confuse AI with AGI.
AI is at least 70 years old – It has been around as a set of principles and computer theory since the 1950s and important components such as neural networks & large language models have been around in one form or another since the 1980s.
Common applications of AI have been around since the turn of the Century – think speech recognition in a car or automated manufacturing robots in a car factory.
So – it’s not new technology, it’s been around for 70 years, and it’s been utilised across many different industries. So, what’s all the fuss about? What is different now? Why are investors flocking to the hot AI startups and hardware companies? Why are governments sounding alarm bells and talking about safeguards? Why are some people claiming human extinction as the next step in AI’s development?
AI Progression over the last decade
The fact is, although AI has been around for a long time, the recent emergence of AI assistants like ChatGPT follows a 5–10-year period of steep investment in so-called model training through machine learning, where the invisible underbelly of the current ’retail’ AI was being built, refined, trained and ultimately turned into what we now call LLMs – Large Language Models.
LLM’s are at the heart of this current AI cycle, and they are bigger than ever before having been brought up on a diet of ‘the internet’ – which now carries mind-boggling amounts of information compared to even 10 years ago. All the big tech platforms have built their own LLM, and many tweaked variants of these now exist (650,000 at the last count) – covering everything from generic text to text (ChatGPT, bard etc.) to text to image & text to video.
All of these large Language Models have certain things in common – they’ve all been trained on masses of data harvested from the internet. Tens of thousands of people have spent millions of hours reading and ‘vetting’ that data before supporting ‘machine learning’ as these models have been refined, and they’ve all benefited from being backed by the richest companies on the planet – Apple, Microsoft, Google, Amazon & Meta.
The use of data in modern AI formats
The existence of massive amounts of training data, coupled with significant advances in processor speeds and capabilities have enabled the real, crucial step-change in AI – a human-like capacity to understand a question and to respond quickly with a sense of comprehension. This advancement effectively means that you don’t need to be a software engineer to generate a response from an LLM.
Even 5 years ago, to harness data results from AI capabilities meant you had to write some code, run it in a complex environment and finesse the results into some useable form – so very much the domain of the IT professionals & scientists. With the wholesale role out of ‘natural language’ assistants – effectively front-ends to these LLMs, (chatGPT, Bard, Claude, GROQ and many, many more), this means that anybody who can read and write can use AI to find stuff out, generate complex learnings and create content – as long as similar stuff was at some point captured on the internet – through a blog, or a forum or a website.
How technology has affected AI enhancements
Another major change that has coincided with the LLM investment is the speed and capability of the silicon processors powering these models. The hardware has got seriously bigger & faster over the last 30 years – and curiously, that has NOT been driven by AI but by the Gaming industry. Computer games have for a long time been one of the big commercial drivers of computer hardware innovation. As game creators come up with bigger more realistic worlds and graphics, the hardware needed to deliver those gaming experiences has had to improve and nowhere has been more important to this trend than the ‘Graphics Card’ and the Graphics Processing Unit (GPU).
Delivering speedy, realistic graphics has been so important to the gaming industry that the concept of moving huge loads of data crunching to a separate device with a bespoke GPU became established almost as soon as the PC did. Once established there has been a 40-year arms race between the graphics card manufacturers to produce the fastest most powerful graphics cards to capture that lucrative gaming market. This has led to innovation in the massive reduction of fabrication scales for GPUs (important to get more oomph in less space) and importantly to the increase in the scale of parallel processing enabled by the underlying silicon chip architecture.
The Role of Parallel Processing
To explain why parallel processing is so important we need to imagine what’s contained in a single-frame image on a monitor– rendering a graphics image onto the screen for just a single frame requires billions of bits arranged in a square the size of the display to represent colour, location and intensity – and those bits need to be ‘transformed’ many times a second to produce the moving image (frames per second). This means that the GPUs need to perform massive parallel computing operations many times a second, and that was what drove the development of these increasingly large parallel processing architectures – specially designed silicon to optimise the crunching of billions of bits in a few milliseconds.
How Crypto Currency influenced AI
Another indication of the unintended wider application of Graphics Card capabilities was with the adoption of GPUs by Bitcoin miners looking to improve the speed of Bitcoin mining 15 years ago. It turned out that the same feature of GPUs that made them so good for graphics was also very effective at solving the proof-of-work problems needed to mine new Bitcoins.
(Sideline…’Proof of work’ is a mathematically complex algorithm that needs to create a requested result before a Bitcoin can be mined. The more bitcoins that are mined, the harder the problem becomes.
That same property of GPUs used for Bitcoin mining is also the same property that is very good at applying the transformation processing required by LLMs and their corresponding AI front ends. Because of this, the dominant Graphics Card and GPU manufacturer – NVIDIA – has seen its stock price increase from under $300 per share to $1,044 in under a year due to the ‘gold rush’ of large tech companies investing so much in their high-end GPUs to build AI datacentres.
In light of this, NVIDIA has re-focused its entire high-end product range & its R&D budget from gaming to AI GPUs. The NVIDIA technology (and their close competitors) is viewed as so fundamental to the future of AI that the US government has banned their export to China. This tells us that the US government at least, believe that AI is here and indeed is the future.
Of course, there’s something of a ‘self-fulfilling prophecy’ about the current AI gold rush – for good or for bad, all that money and focus is going to ensure that AI will become ubiquitous. Whether we like it or not and despite the lack of regulation (or perhaps because of it) – the current trend is unstoppable and that’s as much about the investors as it is about AI itself.
It should also be noted that whatever is known in the public domain, the state-run, military and intelligence domains will already be some years ahead. And it’s easy to see why – there’s such a broad range of existing military capabilities that can be enhanced from better signal filters, better encryption, better automation and optimisation – to things such as drone swarms, autonomous F-16s or independent deadly robots (they do exist in laboratories at least).
What role does AI play in everyday lives?
So that’s all very well you might say – but what does AI do for normal people, doing normal jobs? Can it help businesses? Can it increase productivity? Will it take away my job? Should we be scared?
There is no simple answer to this – the current generative AI has grown huge very quickly and it is essentially version 1 – the implication being it’s at a very early stage of maturity and so is likely to get much more powerful very quickly – I’ll be more interested in version 5 – which is probably 3-5 years away.
In this early stage, there are thousands of AI Assistants and tools covering everything from trivial to deadly – medical research, materials research, chemical research, AI partners (digital girlfriends/boyfriends), voice cloning, image generation, video generation, deep-fakes, cyber-crime support, coding bots, large data analysis, real-time AI content in Ads and so on. But still, the public face of AI such as ChatGPT is still mostly a gimmick – we have yet to see which application of AI will prevail as the AI market has yet to consolidate.
Sure, it’s entertaining and novel, and it can help you write an essay – but as soon as you need to apply it to something difficult & specific – it becomes much harder work to make sure that what is generated is accurate, in context and proportionate to the question. You can soon end up thinking – it’ll be quicker and easier if I just write this or work this out for myself. But many emerging AI tools have been and are being refined and focused on specific tasks or areas of expertise – and here I think is where we will see a much more significant impact in due course.
So, the bottom line, the impact of this AI ‘step-change’ is going to be both positive and negative. AI is not inherently good or bad – it’s a tool that implements and amplifies the intentions (and intelligence or lack of intelligence) of the user of the tool – and that means new kinds of good and bad are going to be enabled by AI. Ultimately, it’s humanity’s job to work out how to deal with it – in much the same way we had to work out how to deal with the internet, and before that the TV, and before that the Radio, and before that the telegraph, and before that the printing press. Each of the above ushered in a step change that had a huge unknown impact on humanity – but hey – we’re still here.
Article Written By: David Hall – Chief Technology Officer – Integrity Communications Group